What do you understand by the expression 'Cluster Analysis'? Explain the difference between hierarchical and non-hierarchical and their pros and cons in legal research.
Find the question and answer of the Research Methodology only on Legal Bites.
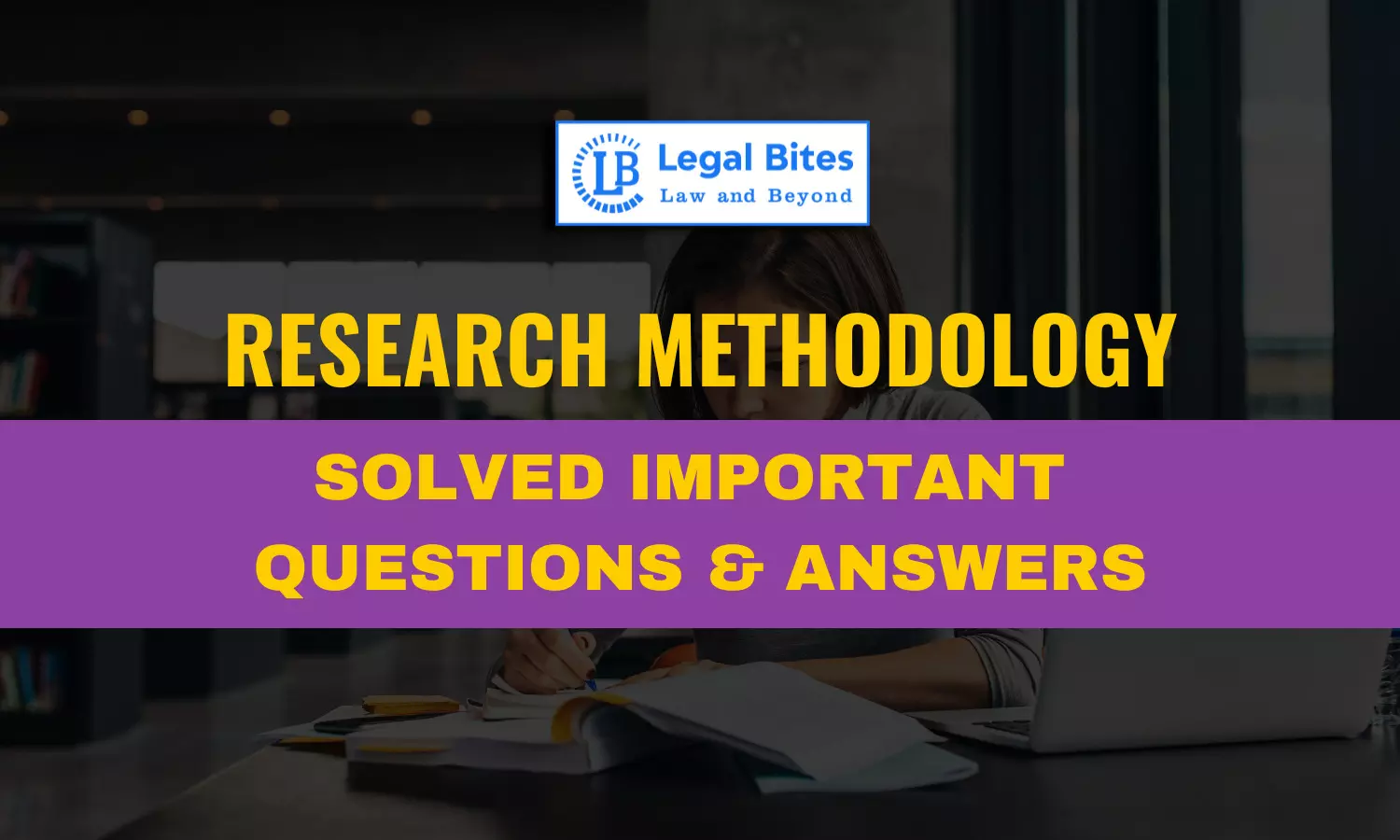
Question: What do you understand by the expression 'Cluster Analysis'? Explain the difference between hierarchical and non-hierarchical and their pros and cons in legal research.Find the question and answer of the Research Methodology only on Legal Bites. [What do you understand by the expression 'Cluster Analysis'? Explain the difference between hierarchical and non-hierarchical and their pros and cons in legal research.]AnswerCluster analysis is a statistical technique used to group...
Question: What do you understand by the expression 'Cluster Analysis'? Explain the difference between hierarchical and non-hierarchical and their pros and cons in legal research.
Find the question and answer of the Research Methodology only on Legal Bites. [What do you understand by the expression 'Cluster Analysis'? Explain the difference between hierarchical and non-hierarchical and their pros and cons in legal research.]
Answer
Cluster analysis is a statistical technique used to group similar objects or entities based on their characteristics or attributes. It is a common data analysis method in various fields, including legal research. The goal of cluster analysis is to identify patterns or relationships within a dataset by organizing the data into meaningful groups, often referred to as clusters.
Hierarchical and non-hierarchical are the two main approaches to cluster analysis.
1. Hierarchical Clustering:
Hierarchical clustering involves creating a hierarchy of clusters, where similar data points are grouped together based on their proximity. It can be further categorized into two types:
Agglomerative: This method starts with each data point as a separate cluster and progressively merges the most similar clusters until all the data points belong to one large cluster. It forms a tree-like structure called a dendrogram, which shows the merging process.
Divisive: This method starts with all data points in one cluster and then iteratively divides the clusters into smaller clusters based on dissimilarity until each data point becomes a separate cluster. Divisive clustering creates a dendrogram as well but in a reverse manner compared to agglomerative clustering.
Pros of hierarchical clustering in legal research:
- Provides a visual representation of the clustering process through dendrograms, aiding in the interpretation and understanding of the relationships between clusters.
- Does not require prior specification of the number of clusters, making it useful when the optimal number of clusters is unknown or ambiguous.
- Allows for exploration at different levels of granularity, enabling researchers to examine both broad and fine-grained patterns.
Cons of hierarchical clustering in legal research:
- Can be computationally intensive and time-consuming, particularly with large datasets.
- May not be suitable for datasets with a high dimensionality or when dealing with large variations in data dissimilarity.
- Difficulties may arise in determining the appropriate level of granularity or cutoff point for dividing the clusters.
2. Non-Hierarchical Clustering:
Non-hierarchical clustering, also known as partitional clustering, involves directly partitioning the data into a predetermined number of clusters without forming a hierarchical structure. It typically requires an initial guess of the number of clusters or relies on optimization algorithms to find an optimal number.
Pros of non-hierarchical clustering in legal research:
- Faster and more efficient than hierarchical clustering, especially for large datasets, as it avoids the iterative merging or division process.
- Allows for greater control over the number of clusters by specifying it in advance or by using optimization techniques.
- Suitable for datasets with high dimensionality and large variations in data dissimilarity.
Cons of non-hierarchical clustering in legal research:
- Requires specifying the number of clusters beforehand, which can be challenging if the optimal number is unknown or subjective.
- May not provide a visual representation of the clustering process, making it less intuitive for interpretation compared to dendrograms.
- Results can vary depending on the initial selection of cluster centres or initial guesses.
In legal research, both hierarchical and non-hierarchical clustering can be valuable research methodologies. The choice between them depends on the specific research objectives, the nature of the dataset, and the available computational resources. Hierarchical clustering can be useful when exploring patterns at different levels of granularity and when the number of clusters is uncertain. On the other hand, non-hierarchical clustering is beneficial when the number of clusters needs to be determined in advance or when efficiency is a priority for larger datasets.
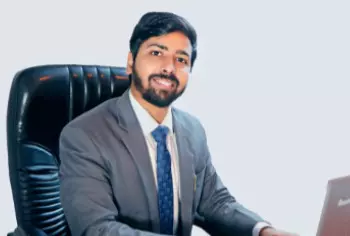
Mayank Shekhar
Mayank is an alumnus of the prestigious Faculty of Law, Delhi University. Under his leadership, Legal Bites has been researching and developing resources through blogging, educational resources, competitions, and seminars.